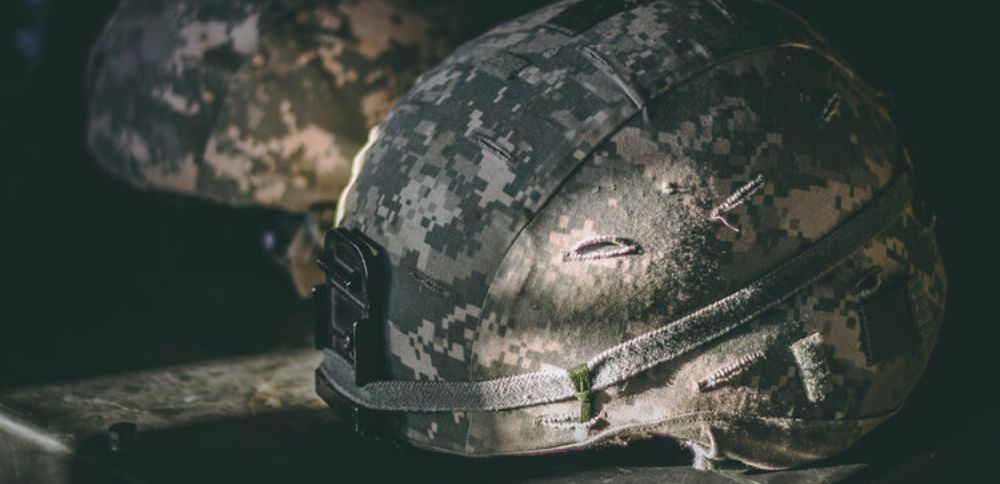
By using state-of-the-art early-warning models, the recent outbreaks of deadly violence in Mali and Ukraine could probably have been predicted.
Violent conflict has escalated recently in Mali.
On January 16, 2020, fourteen villagers were killed by armed men on motorbikes. On Sunday, January 26, suspected Islamist militants killed twenty Malian soldiers in an attack on a military base.
Is there a way to predict, with reasonable accuracy, if and where violent conflict will erupt in the future?
As part of the EU-LISTCO project, the Peace Research Institute Oslo (PRIO) is doing research on subnational early-warning models, using state-of-the-art data and statistical methods. Early-warning systems, such as ViEWS, are systematic procedures comparable to daily weather forecasts set up to provide regular forecasts for conflict-related events. PRIO’s ambition is to make such early-warning insights more accessible and easier to communicate to a broad audience by using visualizations and maps.
The preliminary results of our own model, which combines qualitative and quantitative analysis, suggests that the outbreak of deadly violence in Mali in January 2020 could have been predicted.
The model is based on subnational explanatory variables that previous research on conflict outbreak has found important, such as urban accessibility, distance to international borders, excluded minorities, educational attainment, and gross national income per capita, to name but a few.
Also included are country-level variables such as a liberal democracy index and how much of the country’s territory is under state control—these are expert-coded measures from the V-Dem project.
The goal of our research is to find out whether we can integrate those country-level variables with the slow-moving (structural) subnational variables in order to predict conflicts, and to check the success of such predictions against the actual conflicts that have happened, using high-resolution conflict event data.
If our predictions turn out to be right, this would make future improvements to early-warning models easier and more cost-effective to achieve, as early-warning models would be less dependent on high-resolution data with both temporal and spatial dimensions—which is both costly and time-consuming to collect.
Our preliminary results show that the approach is quite successful, something that bodes well for the prospect of integrating other country-level variables that could capture escalation signals into subnational prediction models.
As highlighted on the map (see figure 1), test predictions conducted for 2016 show several conflict hot spots, such as in Afghanistan, Nigeria, Somalia, and the Great Lakes region of Central Africa, many of which did see conflict in 2016.
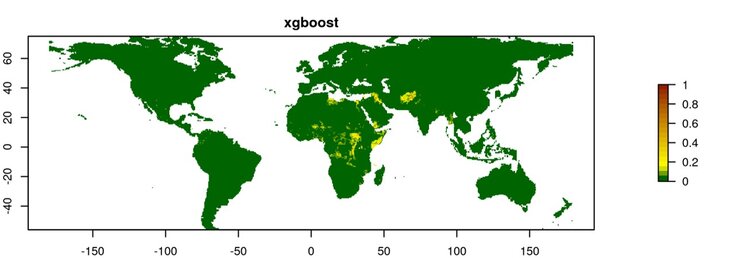
When, for example, comparing our predictions for Mali with actual events in 2016, our predictions are fairly accurate. Most conflict events occurred in and around the central Mopti region (see figure 3), and the prediction map suggests a heightened risk in exactly this area (see figure 2). However, the model does not predict the violence that took place in the northeastern Kidal region.
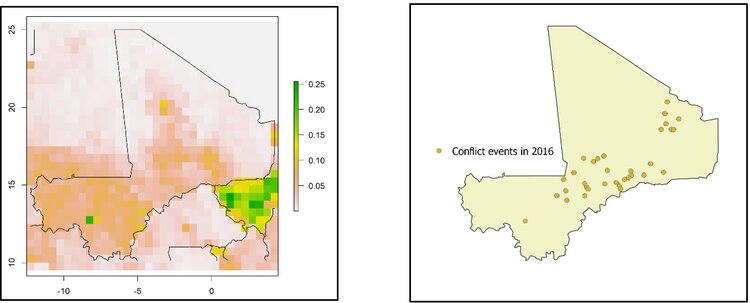
Another example is Ukraine. We see that the prediction model indicates a higher risk of conflict in the eastern parts of Ukraine (see figure 4), and this is also reflected in the map of actual conflict events in 2016 (see figure 5).
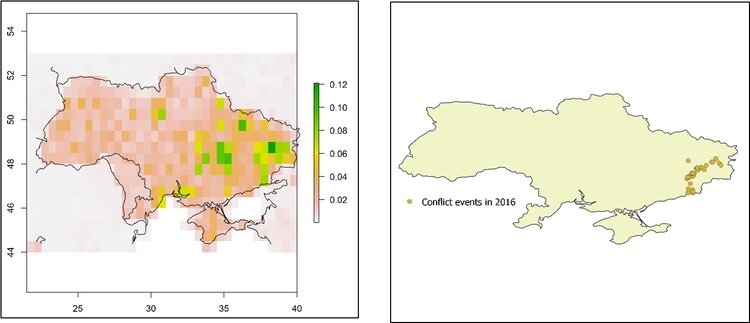
However, there are also conflicts we “mispredict.” A prediction model can make two errors: False negatives, meaning some areas experienced conflict that we fail to predict, and false positives, meaning some conflict predictions did not happen.
Some examples of false negatives in our 2016 predictions include Islamic State cells targeted by the Russian security service in Siberia, Islamic State attacks in Europe, attacks in Algeria by al-Qaeda in the Islamic Maghreb, and some cartel conflicts in Mexico. Mainly terrorist attacks, these are by nature difficult to predict.
On the other hand, the false positives in Guinea, Indonesia, Ivory Coast, Madagascar, Mauritania, and Vietnam are caused by the relatively low subnational human development scores of these countries (measured as life expectancy, income, and schooling). We believe these are countries to pay particular attention to, particularly to understand why armed conflict did not erupt.
Despite the positive results in Mali and Ukraine, the predictions do not pinpoint precisely where conflict will occur, such as in the Mopti region. A critique of these types of forecasting models is that they have limited ability to accommodate context-specific information for each country and conflict dynamics that qualitative research and country experts can provide. To accommodate this critique, we are currently collecting data and redesigning our models to integrate quantitative and qualitative data for forecasting.
As a way to do so, twenty-seven experts have provided their assessment of how conflict will develop in Mali, Georgia, Libya, Tunis, and Ukraine in 2020. Each country expert was asked to draw polygons to indicate where in their country of expertise they expected change in conflict intensity compared to 2019, as well as the degree of this change on a scale of -5 (“much less”) to 5 (“much more”).
As 2020 has just started, it is premature to evaluate these predictions scientifically. However, conflict events in Mali in January 2020 so far confirm the experts’ prediction of increased violence along the border to Burkina Faso, the Ivory Coast, and Niger and decreased violence along the border to Algeria and Mauritania. So far in 2020, conflict events have clustered in the Mopti region where experts predicted an increase. Meanwhile, the predicted increase in violence in the southernmost parts of Mali has not yet materialized (see figures 6 and 7).
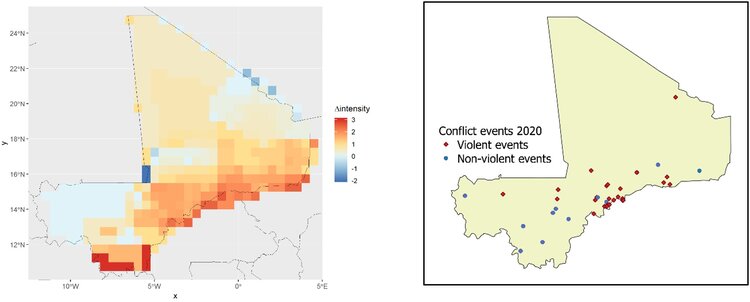
In Ukraine, we see that the experts are expecting violence to increase primarily in previous conflict areas and decrease south of Odessa in the border areas to Moldova and along the border to Hungary in the west. Also, the experts anticipate more violence in the areas bordering Russia (see figure 8).
The violence on the Russian borders has not materialized yet, nor in Crimea, but there are continuous attacks along the established battle-lines in the Donbas region (see figure 9).
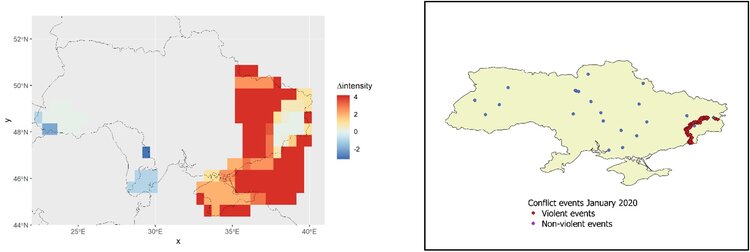
Once final conflict event data for 2020 is released, the experts’ predictions can be properly evaluated and compared to the actual conflict areas, and we can examine how to integrate their predictions into our quantitative model. So far, the results of the predictions present an auspicious approach to future subnational early warning of conflict.
Now what does this mean for policymakers?
By indicating where in a country the risk of conflict is higher, we can direct our attention accordingly and focus toward specific geographical areas, like in Mali’s Mopti region in January 2020.
While this is just one tool out of many that should feed into foreign offices and NGOs to help them improve their conflict prevention measures, it has the potential to become an important one.
Håvard Mokleiv Nygård is the Research Director of the Peace Research Institute Oslo. Siri Aas Rustad, Andreas Forø Tollefsen, and Jonas Vestby are Senior Researchers at the Peace Research Institute Oslo.
This blog post was originally published on Carnegie Europe’s Strategic Europe blog.
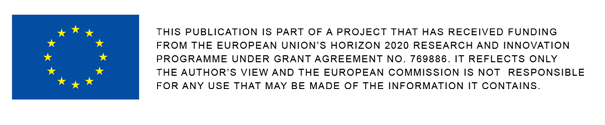